Introduction
This research focuses mainly on the automated classification of pollen using computer vision and machine learning. Classification of pollen is important because it can be used to warn allergy sufferers in period of elevated risk; it also has practical applications in agriculture for ensuring effective pollination of food crops. Despite the numerous applications, manual classification of pollen is tedious and must be performed by highly skilled specialists. Current automated methods still require a large number of training samples per pollen type, which may grow as the classifier is trained to distinguish more and more different types. We reduce the training effort of the classification with three main key points:
- The transfer of classification rules from existing pollen types to new types
- The introduction of an active selection criterion to obtain valuable training samples
- The development of a new feature, spike count
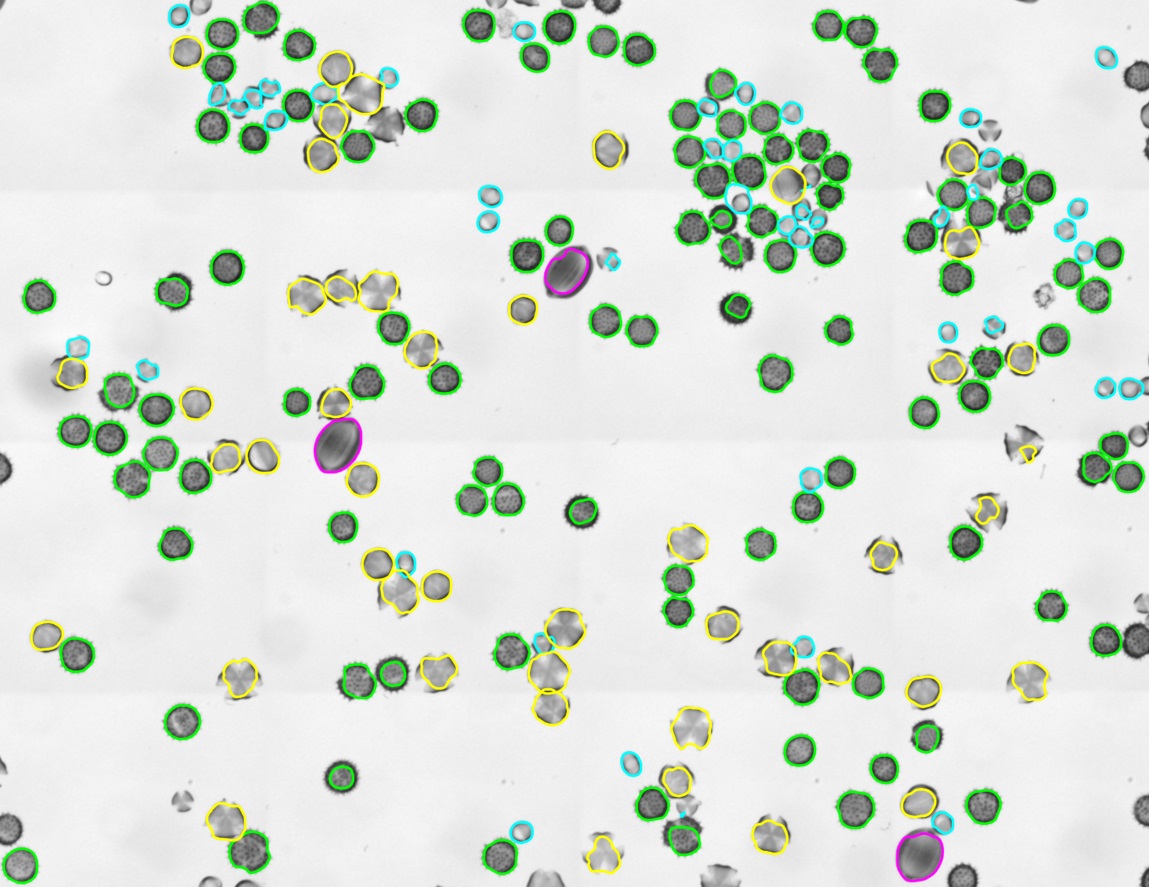
Publication
People

N. Rich Nguyen, MSc PhD Student
CS Department, UNC Charlotte Phone: (704) 687-8582
Office: 404 WOODWARD Email: nhnguye1 at uncc dot
edu

Matina Donaldson-Matasci, PhD Post-doctoral Researcher
Biology Department, University of Arizona Phone: n/a
Office: Tucson, Arizona Email: matina.at.email.dot.arizona.dot.edu

Min Shin, PhD Associate Professor CS Department, UNC Charlotte Phone: (704) 687-8578
Office: 403D WOODW Email: mcshin at uncc dot
edu